AI health tools go untested in many U.S. hospitals: study
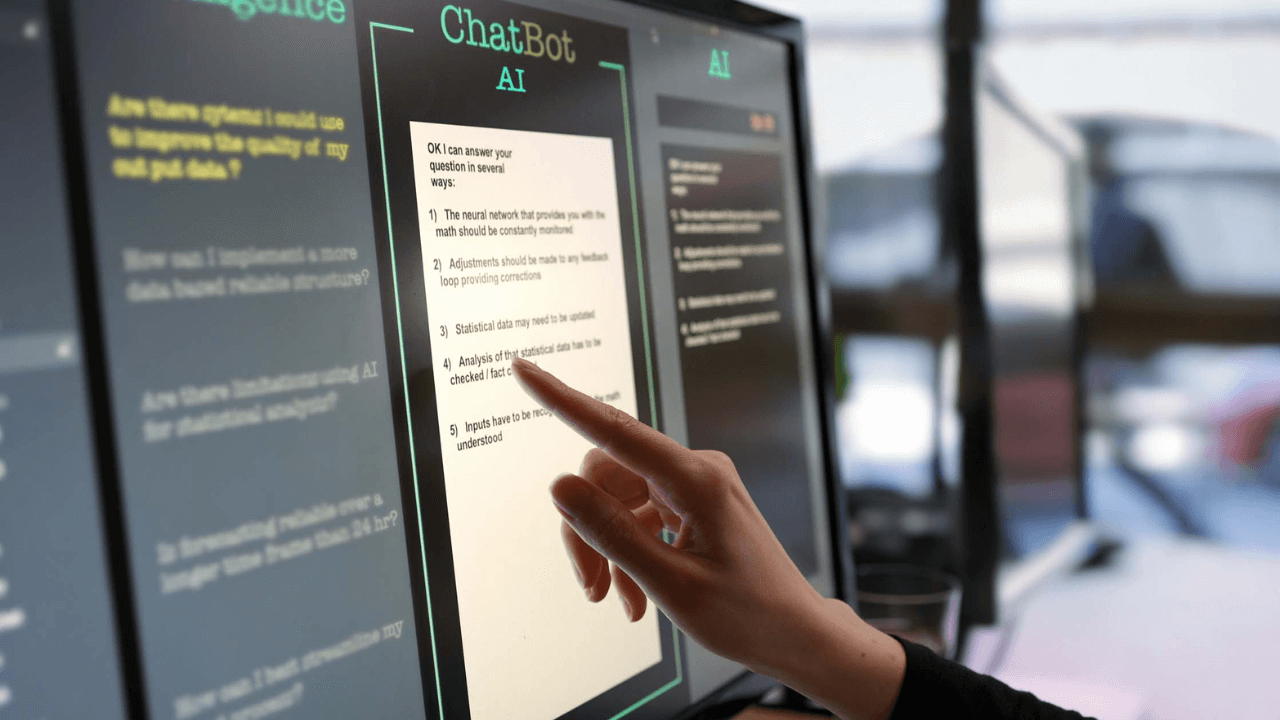
WASHINGTON, D.C., UNITED STATES — A recent study published in Health Affairs revealed that while 65% of U.S. hospitals use artificial intelligence (AI) or predictive models, only 61% evaluate these tools for accuracy using local data, and a mere 44% assess them for bias.
This lack of scrutiny raises concerns about patient safety and equitable care, particularly in under-resourced hospitals.
The study analyzed data from 2,425 hospitals nationwide and found that predictive models are most commonly used to forecast inpatient health trajectories (92%) and identify high-risk outpatients (79%).
However, administrative applications like billing automation (36%) are less frequently evaluated locally, reflecting a potential underestimation of risks associated with non-clinical AI tools.
Resource disparities widen the digital divide
Hospitals with greater financial resources and technical expertise—such as academic medical centers—are more likely to develop their own AI models and conduct local evaluations. Conversely, rural and critical-access hospitals often rely on pre-built algorithms from electronic health record (EHR) vendors, which may not align with their patient populations.
“By focusing on the differences in evaluation capacity among hospitals, this research highlights the risks of a growing digital divide between hospital types, which threatens equitable treatment and patient safety,” said Paige Nong, lead author and assistant professor at the University of Minnesota’s School of Public Health.
The study also noted that vendor-developed models dominate the market, with 79% of hospitals using algorithms from their EHR providers. Hospitals developing their own models were significantly more likely to evaluate them for both accuracy and bias.
Bias in AI exacerbates health inequities
Experts warn that algorithmic bias can worsen healthcare disparities by perpetuating inequities in care delivery. For instance, biased models may underrepresent marginalized populations or fail to account for socioeconomic factors, leading to misdiagnoses or inadequate treatment plans.
“Bias is a central focus,” the researchers wrote, emphasizing that unchecked algorithms could harm vulnerable patient groups by creating barriers to care.
Call for policy interventions
To address these issues, the researchers recommend policies requiring AI developers to provide greater transparency about their algorithms. They also urge targeted interventions to help under-resourced hospitals evaluate their tools effectively. Without such measures, the “rich-get-richer” effect in healthcare could deepen.
“Many better-funded hospitals can design their own models tailored to their patients,” Nong explained. “In contrast, critical-access hospitals are buying these products ‘off the shelf,’ which can mean they’re designed for a patient population that may look very different from their actual patients.”
This study underscores the urgent need for standardized evaluation practices to ensure AI tools benefit all patients equally, regardless of hospital resources or location.